Hello!
It’s a clear-skied, crisp day here in Minnesota. Winter is coming, but not today.
While we wait for the world to turn and the seasons to change, why not pass some time thinking about database configuration?
I recently moved from SQLite to PostgreSQL as the database for pythondocs.xyz, my project that tries to bring a Google-like experience to Python’s official documentation. (The main motivation was to improve search results with Postgres’ full-text search capabilities.)
Swapping the DB engines proved to be remarkably straightforward: the SQLAlchemy ORM abstracted away dialect differences, and I was already using an abstract Database class with FastAPI, so I just needed to write another implementation.
Keep reading for a walkthrough of the code…
Pre-requisites
You need Postgres running on your machine or in a Docker container.
I’m using Docker Compose for this project. I won’t explain more today but I’m happy to make it the subject of a future blog post if people are interested?
You also need these Python packages:
- uvicorn (web server), python-dotenv (settings loader), fastapi (web framework), sqlalchemy (ORM) , asyncpg (database driver)
I’m presenting this example as a single Python file so that it’s easy to copy and understand. But I’ll also mention original file paths, as you’ll definitely want more organization in a real project.
Here are the imports for the example:
import os
from abc import ABC, abstractmethod
from typing import AsyncIterator, Optional
import uvicorn
from dotenv import load_dotenv
from fastapi import Depends, FastAPI
from fastapi.responses import JSONResponse
from sqlalchemy import select
from sqlalchemy.ext.asyncio import AsyncSession, create_async_engine
from sqlalchemy.orm import sessionmaker
# not included in example
from app.models import Folder
Config elation
The config module provides production or development settings, depending on environment variable. It also loads the Postgres password from an .env file. The idea is that you add .env to your .gitignore file so that you don’t mix secrets with the rest of your code.
# config.py
load_dotenv()
class Config(ABC):
POSTGRES_USERNAME = "postgres"
POSTGRES_DB_NAME = "postgres"
# localhost for development purposes
POSTGRES_HOST = "localhost"
POSTGRES_PORT = "5432"
# password stored in .env file
POSTGRES_PASSWORD = os.getenv("POSTGRES_PASSWORD")
SQL_COMMAND_ECHO = False
class DevelopmentConfig(Config):
SQL_COMMAND_ECHO = True
class ProductionConfig(Config):
# hostname in Docker network for production
POSTGRES_HOST = "db"
def get_config() -> Config:
env = os.getenv("ENV")
if env == "development":
return DevelopmentConfig()
return ProductionConfig()
config = get_config()
Data based
Here, we define an abstract Database class, with a __call__() method that works with FastAPI’s dependency injection. Its setup() method is provided in concrete implementations, like PostgresDatabase.
# database/base.py
class Database(ABC):
def __init__(self):
self.async_sessionmaker: Optional[sessionmaker] = None
async def __call__(self) -> AsyncIterator[AsyncSession]:
"""For use with FastAPI Depends"""
if not self.async_sessionmaker:
raise ValueError("async_sessionmaker not available. Run setup() first.")
async with self.async_sessionmaker() as session:
yield session
@abstractmethod
def setup(self) -> None:
...
# database/postgres.py
def get_connection_string(driver: str = "asyncpg") -> str:
return f"postgresql+{driver}://{config.POSTGRES_USERNAME}:{config.POSTGRES_PASSWORD}@{config.POSTGRES_HOST}:{config.POSTGRES_PORT}/{config.POSTGRES_DB_NAME}"
class PostgresDatabase(Database):
def setup(self) -> None:
async_engine = create_async_engine(
get_connection_string(),
echo=config.SQL_COMMAND_ECHO,
)
self.async_sessionmaker = sessionmaker(async_engine, class_=AsyncSession)
Stitch it all together
There are a few things going on here:
- An instance of PostgresDatabase is created in the depends module.
- The fast_api object is created in main.
- db.setup() is run as a FastAPI startup event only. This means that all code files can be safely imported without side effects.
- A route is definied which performs a simple DB query using FastAPI’s Depends.
- Finally, the uvicorn web server is started.
# depends.py
db = PostgresDatabase()
# main.py
fast_api = FastAPI()
@fast_api.on_event("startup")
async def setup_db() -> None:
db.setup()
# routes.py
@fast_api.get("/example/")
async def db_query_example(
session: AsyncSession = Depends(db),
) -> JSONResponse:
results = await session.execute(select(Folder))
return results.all()
# run_uvicorn.py
if __name__ == "__main__":
uvicorn.run(
"fastapi_postgres_async_example:fast_api",
)
That’s it!
If the computer Gods smile upon you, you now have a working – and pretty fast – database configuration.
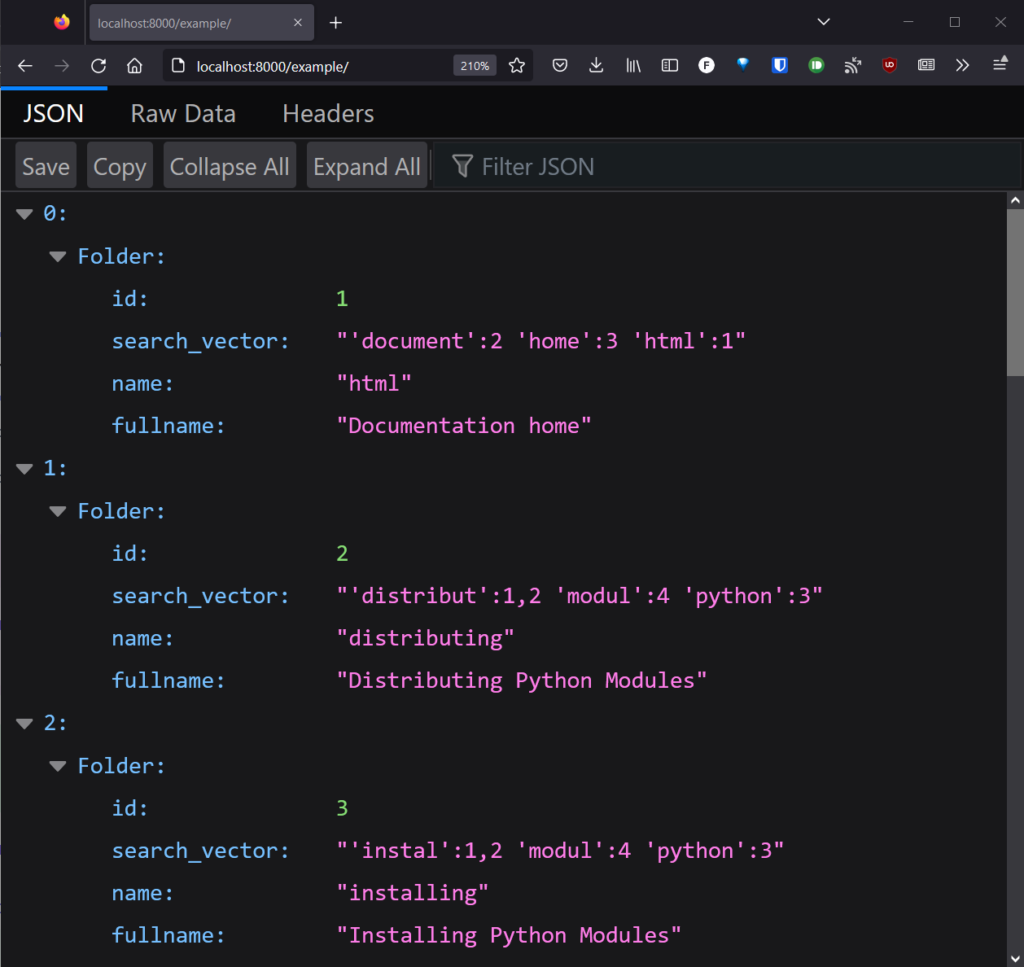
Thanks for reading! I hope this has been useful to someone. Please let me know in the comments.